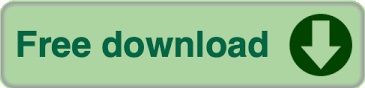
Its use should be encouraged in modern epidemiologic teaching and practice.Īverage treatment effects on the treated (ATT) Average treatment effects on the untreated (ATU) G-computation Parametric g-formula Resampling Simulation. Rohen Shah explains the vocabulary behind the treatment effects literature, describing the average treatment effect (ATE), the ATT, ATN, ITT, and LATE. The g-computation algorithm is a powerful way of estimating standardized estimates like the ATT and ATU. In our illustrative example, the effect (risk difference ) of a higher education on angina among the participants who indeed have at least a high school education (ATT) was -0.019 (95% CI: -0.040, -0.007) and that among those who have less than a high school education in India (ATU) was -0.012 (95% CI: -0.036, 0.010). The estimates for ATT, ATU and average treatment effect (ATE) were of similar magnitude, with ATE being in between ATT and ATU as expected. To obtain marginal effect estimates for ATT and ATU we used a three-step approach: fitting a model for the outcome, generating potential outcome variables for ATT and ATU separately, and regressing each potential outcome variable on treatment intervention. In this paper we illustrate the steps for estimating ATT and ATU using g-computation implemented via Monte Carlo simulation. A closed-form locally semiparametric efficient estimator is obtained in the simple case of binary IV and outcome and the efficiency bound is derived for the more general case.Ĭounterfactuals Double robustness Effect of treatment on the treated Instrumental variable Unmeasured confounding.Average treatment effects on the treated (ATT) and the untreated (ATU) are useful when there is interest in: the evaluation of the effects of treatments or interventions on those who received them, the presence of treatment heterogeneity, or the projection of potential outcomes in a target (sub-) population. For inference, we propose three different semiparametric approaches: (i) inverse probability weighting (IPW), (ii) outcome regression (OR), and (iii) doubly robust (DR) estimation, which is consistent if either (i) or (ii) is consistent, but not necessarily both. In this paper, we present a novel framework for identification and inference using an IV for the marginal average treatment effect amongst the treated (ETT) in the presence of unmeasured confounding. The instrumental variable (IV) design plays the role of a quasi-experimental handle since the IV is associated with the treatment and only affects the outcome through the treatment. In observational studies, treatments are typically not randomized and therefore estimated treatment effects may be subject to confounding bias.
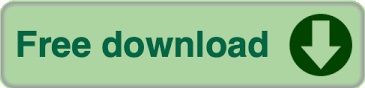